Colonoscopy is a widely used medical procedure to examine the inside of the colon and rectum. It is typically performed to diagnose and treat a variety of conditions, including colorectal cancer, polyps, and inflammatory bowel disease. The traditional approach to colonoscopy involves the use of a passive learning template, where the endoscopist relies solely on their own expertise to identify and interpret findings.
However, recent advances in medical technology have introduced the concept of active learning template diagnostic procedure colonoscopy. This technique utilizes artificial intelligence (AI) algorithms to provide real-time guidance to the endoscopist during the procedure. The AI is trained on a vast database of colonoscopy images, allowing it to recognize and highlight suspicious lesions and other abnormalities with high accuracy.
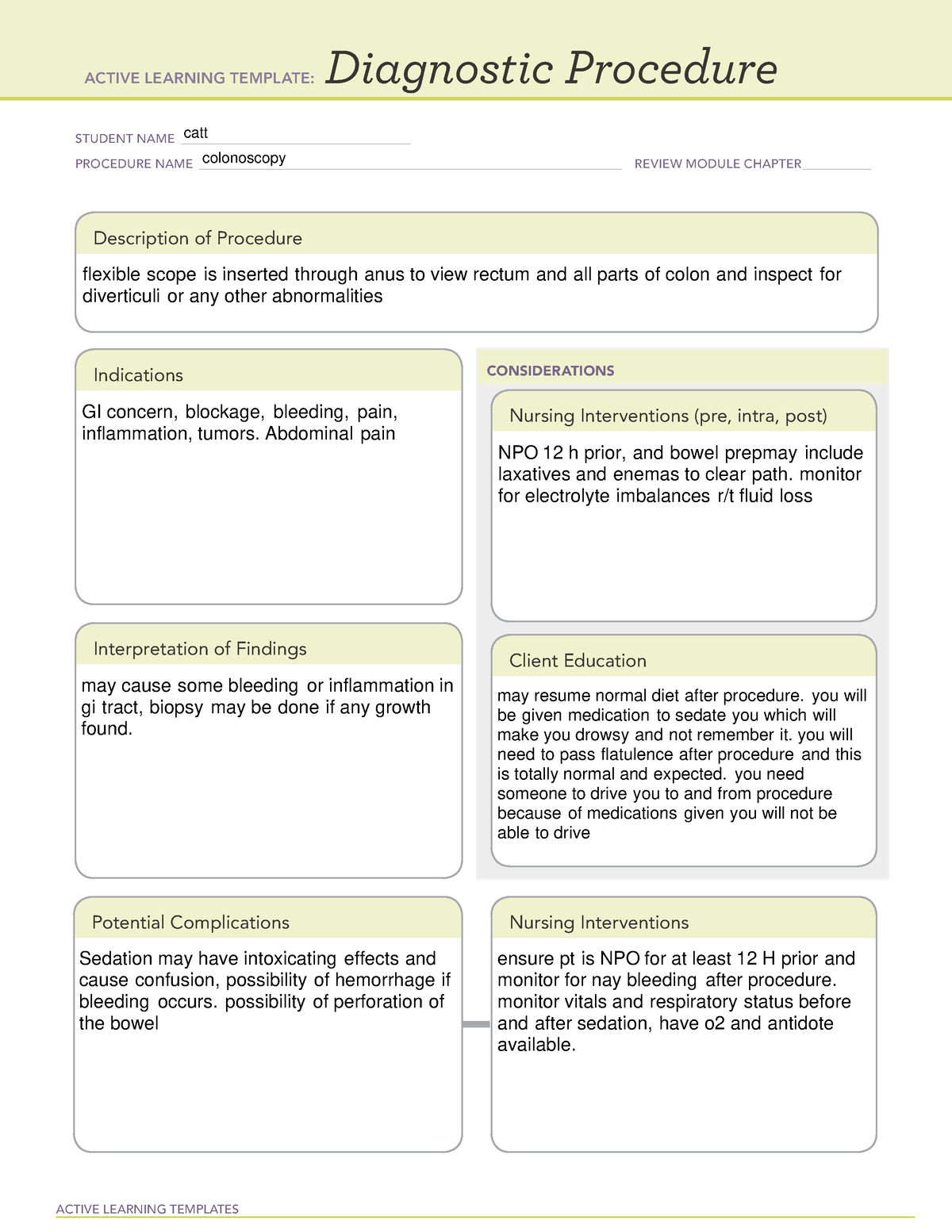
Enhanced Diagnostic Capabilities
Active learning template diagnostic procedure colonoscopy offers several advantages over the traditional passive learning template approach. By leveraging AI, the technique significantly enhances the diagnostic capabilities of endoscopists. The AI can detect lesions that are difficult to identify with the naked eye, such as flat or serrated polyps that may be precursors to cancer. This improved detection rate leads to earlier diagnosis and treatment, which can have a profound impact on patient outcomes.
In addition to enhancing lesion detection, active learning template diagnostic procedure colonoscopy also aids in the characterization of lesions. The AI can provide information about the size, shape, texture, and vascularity of lesions, helping the endoscopist determine the likelihood of malignancy. This real-time feedback allows for more informed decision-making during the procedure, reducing the need for unnecessary biopsies and ensuring that appropriate interventions are taken promptly.
Improved Accuracy and Efficiency
Active learning template diagnostic procedure colonoscopy has been shown to significantly improve both the accuracy and efficiency of colonoscopy. By providing real-time guidance, the AI helps endoscopists identify and interpret findings more accurately, leading to a reduction in false positives and false negatives. This improved accuracy contributes to better patient outcomes by ensuring that appropriate treatment decisions are made for each individual.
Furthermore, the efficiency of colonoscopy is enhanced by the use of active learning templates. The AI can quickly and efficiently scan the colonoscopic images, highlighting suspicious areas that require further attention. This allows the endoscopist to focus their efforts on the areas that are most likely to yield diagnostic information, reducing the time required for the procedure and making it a more comfortable experience for patients.
Conclusion
Active learning template diagnostic procedure colonoscopy represents a significant advancement in the field of colonoscopy. By leveraging AI, the technique enhances the diagnostic capabilities of endoscopists, improves the accuracy and efficiency of the procedure, and ultimately leads to better patient outcomes. As the technology continues to develop, the impact of active learning templates on colonoscopy is expected to grow even more profound, further improving the quality of care for patients undergoing this essential procedure.